Predictive maintenance has emerged as a game-changer in industrial operations that allows businesses to anticipate and address equipment failures before they occur. By harnessing the power of advanced analytics, sensor data, and machine learning algorithms, organizations can optimize maintenance schedules, reduce downtime, and extend the lifespan of critical assets. In this article, we will explore benefits, implementation strategies, and frequently asked questions about this approach.
What is Predictive Maintenance?
It is a proactive maintenance strategy that relies on data-driven insights to predict equipment failures before they happen. Organizations can schedule maintenance activities precisely when needed by monitoring the condition of machinery in real time. This avoids unnecessary downtime and reduces operational costs.
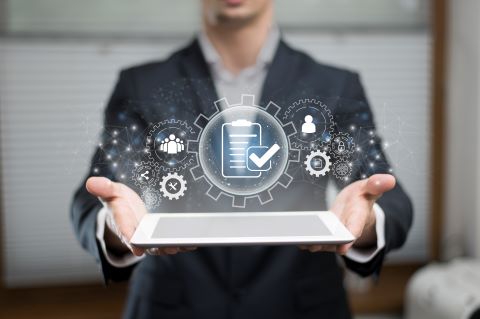
Predictive Maintenance Vs Preventive Maintenance
Preventive maintenance schedules maintenance tasks regularly regardless of the actual condition of the equipment. However, predictive maintenance leverages data and analytics to determine the optimal time for maintenance based on the real-time health of the machine. This difference allows organizations to adopt a targeted and cost-effective maintenance strategy.
The Evolution of Maintenance Strategies
Historically, maintenance strategies have evolved from reactive (where repairs are made after an equipment failure) to preventive maintenance. Predictive maintenance takes this evolution further by leveraging technology to forecast potential failures, enabling organizations to address issues before they disrupt operations.
Benefits of Predictive Maintenance
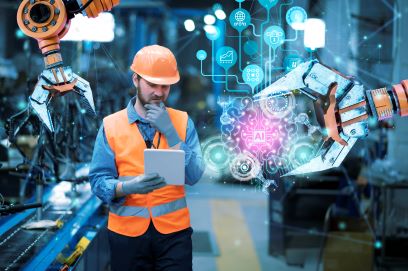
Increased Equipment Reliability
By identifying and addressing potential issues before they escalate, organizations can ensure that their machinery operates at peak performance, reducing the risk of unexpected failures.
Reduced Downtime
It minimizes unplanned downtime by scheduling maintenance activities during planned production breaks or low-demand periods. This targeted approach ensures that maintenance interventions are performed when they are most convenient, reducing disruptions to operations.
Cost Savings
Implementing this system can lead to significant cost savings over time. Companies can optimize their maintenance budgets and allocate resources more efficiently.
Extended Equipment Lifespan
Proactively addressing issues as they arise can contribute to extending the lifespan of equipment. It helps organizations maximize the return on investment in their assets by preventing premature wear and tear and ensuring that equipment operates within optimal parameters.
Improving Safety and Compliance
By ensuring that equipment is always in top condition, organizations can minimize the risk of accidents and regulatory non-compliance, fostering a safer working environment.
How to implement Predictive Maintenance
Data Collection
Effective predictive maintenance relies on the collection of relevant data from sensors installed on critical tools. These sensors monitor various parameters such as temperature, vibration, and fluid levels, providing a real-time snapshot of machine health.
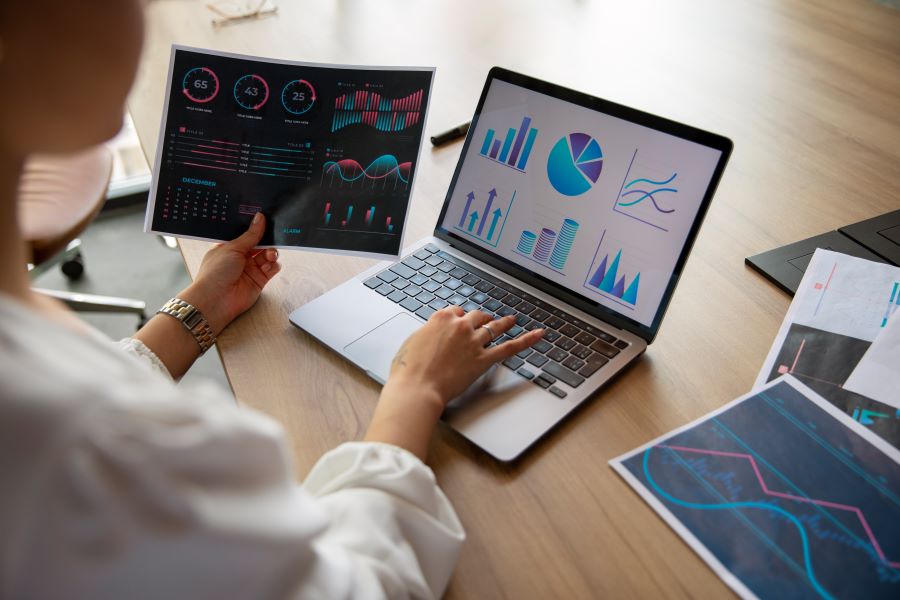
Choosing the Right Sensors
Selecting the appropriate sensors is a critical aspect of implementing predictive maintenance. Different types of machines may require different sensors to capture relevant data correctly. Understanding the specific needs of each asset is crucial to building a robust system.
Data Analysis and Machine Learning
Once the data is collected, sophisticated algorithms and machine learning models analyze the information to identify patterns and anomalies. These models can predict when a machine is likely to fail, providing valuable insights that inform maintenance decisions.
Building a Predictive Maintenance Team
This approach requires a multidisciplinary team that includes data scientists, maintenance engineers, and IT professionals. Collaboration between these teams is essential for successful implementation, ensuring that the system aligns with both technical and operational requirements.
Overcoming Implementation Challenges
While the benefits are substantial, implementation may pose challenges. Common issues include data quality, integration with existing systems, and organizational resistance to change. Addressing these challenges proactively is crucial for the successful deployment of predictive maintenance.
How does predictive maintenance work?
It works by leveraging data, sensors, and advanced analytics to predict when equipment is likely to fail, enabling organizations to perform maintenance activities proactively. Here’s a step-by-step breakdown:
Data Collection
- Sensor Installation: Sensors are strategically installed on critical equipment. These sensors monitor various parameters, such as temperature, vibration, pressure, fluid levels, and other relevant factors depending on the type of machinery.
- Continuous Data Gathering: Sensors continuously collect real-time data from the machine during regular operation. This data provides insights into the current health and performance of the machinery.
Data Processing and Storage:
- Data Transmission: The data collected by sensors is transmitted to a central system for processing. In some cases, edge computing is employed to process data closer to the source, reducing latency and allowing for faster decision-making.
- Data Storage: Processed data is stored in databases, allowing for historical analysis and the development of trends over time. This historical data is crucial for training machine learning models.
Data Analysis and Machine Learning:
- Pattern Recognition: Advanced analytics, including machine learning algorithms, are applied to the collected data. These algorithms analyze patterns and correlations within the data to identify normal operating conditions and detect anomalies.
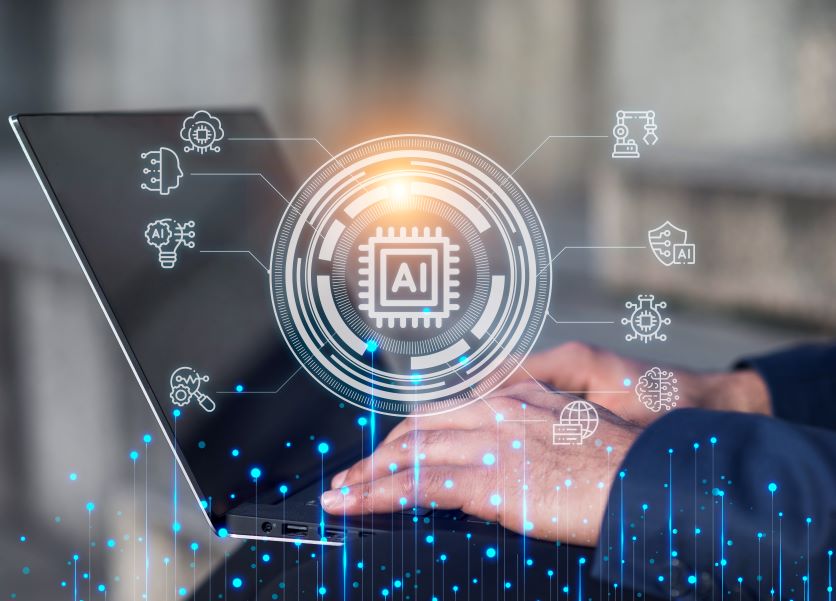
Also read, how to use Natural Language Processing (NLP) for better AI.
- Model Training: Machine learning models are trained using historical data to understand the relationship between sensor readings and impending equipment failures. The more accurate the training data and the more sophisticated the algorithms, the better the predictive capabilities of the system.
Predictive Modeling
- Failure Prediction: Once the machine learning models are trained, they can predict when equipment is likely to fail. The system considers deviations from normal operating conditions, trends in sensor data, and any warning signs that precede failures.
- Risk Assessment: Predictive maintenance systems often provide a risk assessment, indicating the likelihood and severity of a potential failure. This information helps maintenance teams prioritize and plan their activities.
Decision Support:
- Alerts and Notifications: When this system identifies a potential issue, it generates alerts and notifications. These alerts can be sent to maintenance personnel, triggering a response to address the predicted problem.
- Optimal Scheduling: Predictive maintenance systems not only predict failures but also recommend the optimal time for maintenance activities. This allows organizations to schedule maintenance during planned downtimes, minimizing the impact on operations.
Implementation of Maintenance Activities
- Proactive Maintenance: Armed with predictive insights, maintenance teams can proactively address potential issues. This may involve replacing worn-out components, conducting repairs, or performing other measures to avoid unexpected failures.
- Optimization of Resources: It helps organizations optimize their use of resources by directing maintenance efforts only where and when they are needed. This contrasts with traditional preventive maintenance, which relies on fixed schedules.
Continuous Improvement
- Feedback Loop: Predictive maintenance systems benefit from a continuous feedback loop. As new data is collected, it is used to refine and improve the accuracy of the machine learning models. This iterative process ensures that the system adapts to changes in equipment behavior over time.
- Performance Monitoring: Organizations monitor the performance of the system to assess its effectiveness. Key performance indicators (KPIs) such as reduced downtime, cost savings, and extended equipment lifespan are used to measure the success of the predictive maintenance program.
By combining real-time data, machine learning, and proactive decision-making, predictive maintenance empowers organizations to move away from reactive maintenance practices, reduce downtime, and optimize the performance of their critical assets.
Future Trends in Predictive Maintenance
Integration with IoT and Edge Computing
This integration (IoT and edge computing) enables faster data processing and decision-making at the edge of the network, reducing latency and improving the responsiveness of systems.
Artificial Intelligence Advancements
As artificial intelligence (AI) continues to advance, predictive maintenance models are expected to become more sophisticated. AI algorithms can analyze complex patterns and correlations within data, further improving the accuracy of predictions and reducing the occurrence of false positives.
Cloud-based Solutions
The adoption of cloud-based solutions is on the rise, allowing companies to access and analyze data from anywhere. Cloud platforms provide scalability, flexibility, and collaboration capabilities, making it easier to deploy and manage predictive maintenance systems.
Read more, 9 types of cloud computing services to watch out for.
In conclusion, the successful implementation of predictive maintenance requires a holistic approach that encompasses data collection, analysis, organizational collaboration, and ongoing optimization. As organizations embrace this proactive maintenance strategy, they position themselves to not only maximize the efficiency and reliability of their equipment but also stay ahead in an increasingly competitive and dynamic business landscape.